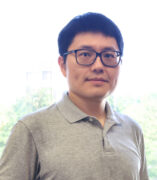
Wenxin Zhou
Associate Professor
Department of Information and Decision Sciences
Pronouns: He/Him/His
Contact
Building & Room:
UH 2423
Address:
601 S. Morgan St., Chicago, IL 60607
Office Phone:
Email:
CV Link:
Related Sites:
About
My general research interests range from parametric to nonparametric regression problems, including high-dimensional regression under sparsity, low-rank matrix recovery/estimation, kernel methods, and deep neural networks for nonparametric regression. More recently, I have focused on tail-centric regressions, such as quantile and expected shortfall regression. I am also interested in developing privacy preserving statistical methods and tail-robust techniques for regression and factor models.
Selected Grants
NSF Division of Mathematical Sciences Award, NSF-DMS 2113409, 2021–2025, PI
Australian Research Council Discovery Project, DP230100147, 2023–2026, Partner Investigator (PI: Aurore Delaigle)
NSF Division of Mathematical Sciences Award, NSF-DMS 1811376, 2018–2021, PI
UCSD, Hellman Fellowship, 2020-2021, PI
Selected Publications
- S. Zhang, X. He, K. M. Tan and W.-X. Zhou. (2025). High-dimensional expected shortfall regression. Journal of the American Statistical Association, in press. [DOI:10.1080/01621459.2024.2448860].
- M. Yu, Y. Wang, S. Xie, K. M. Tan and W.-X. Zhou. (2025). Estimation and inference for nonparametric expected shortfall regression over RKHS. Journal of the American Statistical Association, in press [DOI:10.1080/01621459.2024.2441657].
- T. Zhao, W.-X. Zhou and L. Wang. (2025). Private optimal inventory policy learning for feature-based newsvendor with unknown demand. Management Science, in press. [DOI:10.1287/mnsc.2023.01268].
- J. Fan, Y. Gu and W.-X. Zhou. (2024). How do noise tails impact on deep ReLU networks? The Annals of Statistics, 52(4): 1845-1871.
- X. He, K. M. Tan and W.-X. Zhou. (2023). Robust estimation and inference for expected shortfall regression with many regressors. Journal of the Royal Statistical Society: Series B, 85(4):1223-1246.
- He, X., Pan, X., Tan, K. M. and Zhou, W.-X. (2023). Smoothed quantile regression with large-scale inference. Journal of Econometrics, 232(2): 367-388.
- Tan, K. M., Wang, L. and Zhou, W.-X. (2022). High-dimensional quantile regression: Convolution smoothing and concave regularization. Journal of the Royal Statistical Society, Series B, 84(1): 205-233.
- X. He, X. Pan, K. M. Tan and W.-X. Zhou. (2022). Scalable estimation and inference for censored quantile regression process. The Annals of Statistics, 50(5): 2899-2924.
- K. M. Tan, H. Battey and W.-X. Zhou. (2022). Communication-constrained distributed quantile regression with optimal statistical guarantees. Journal of Machine Learning Research, 23(272): 1-61.
- Q. Sun, W.-X. Zhou and J. Fan. (2020). Adaptive Huber regression. Journal of the American Statistical Association, 115(529): 254-265.
- X. Chen and W.-X. Zhou. (2020). Robust inference via multiplier bootstrap. The Annals of Statistics, 48(3): 1665-1691.
- J. Fan, Y. Ke, Q. Sun and W.-X. Zhou. (2019). FarmTest: Factor-adjusted robust multiple testing with approximate false discovery control. Journal of the American Statistical Association, 114(528): 1880-1893.
- W.-X. Zhou, K. Bose, J. Fan and H. Liu. (2018). A new perspective on robust M-estimation: Finite sample theory and applications to dependence-adjusted multiple testing. The Annals of Statistics, 46(5): 1904-1931.
- J. Fan, Q.-M. Shao and W.-X. Zhou. (2018). Are discoveries spurious? Distributions of maximum spurious correlations and their applications. The Annals of Statistics, 46(3): 989-1017.
- E. X. Fang, H. Liu, K.-C. Toh and W.-X. Zhou. (2018). Max-norm optimization for robust matrix recovery. Mathematical Programming, Series B, 167, 5-35.
- J. Fan and W.-X. Zhou. (2016). Guarding against spurious discoveries in high dimensions. Journal of Machine Learning Research, 17(203): 1-34.
- A. Delaigle, P. Hall and W.-X. Zhou. (2016). Nonparametric covariate-adjusted regression. The Annals of Statistics, 44(5): 2190-2220.
- J. Chang, Q.-M. Shao and W.-X. Zhou. (2016). Cramér-type moderate deviations for Studentized two-sample U-statistics with applications. The Annals of Statistics, 44(5): 1931-1956.
- A. Delaigle and W.-X. Zhou. (2015). Nonparametric and parametric estimators of prevalence from group testing data with aggregated covariates. Journal of the American Statistical Association, 110(512): 1785-1796.
- Q.-M. Shao and W.-X. Zhou. (2014). Necessary and sufficient conditions for the asymptotic distributions of coherence of ultra-high dimensional random matrices. The Annals of Probability, 42(2): 623-648.
- T. T. Cai and W.-X. Zhou. (2013). A max-norm constrained minimization approach to 1-bit matrix completion. Journal of Machine Learning Research, 14(78): 3619-3647.
Publication Aggregators
Service to Community
- Associate Editor, The Annals of Statistics (2022-2024)
- Associate Editor, Statistics: A Journal of Theoretical and Applied Statistics (2020–2023)
- Reviewers for OR, AOS, AOP, JRSS-B, JASA, Biometrika, JMLR, JOE, JBES, IEEE-TIT, IAI, AIHP, Statistical Science, JCGS,Bernoulli, EJS, ET, etc
Professional Leadership
Associate Editor, The Annals of Applied Probability (01/2022–Present)
Associate Editor, Journal of the Royal Statistical Society: Series B (01/2022–Present)
Education
Ph.D. in Mathematics (Specializing in Statistics) – Hong Kong University of Science and Technology, 2013
B.Sc. in Mathematics – Shanghai Jiao Tong University, 2008
Research Currently in Progress
Empowering Climate Science through Effective Tail Learning Methodology and Theory
Intellectual Property
Python package 'quantes':
Joint Quantile and Expected Shortfall Regression (https://pypi.org/project/quantes/)
R package 'conquer':
Convolution-Smoothed Quantile Regression (https://cran.r-project.org/web/packages/conquer/index.html)